Modeling spatial distribution of internal corrosion
in API 5L pipes carrying gasohol
This research paper was presented at CORCON, the annual conference and expo on corrosion science and engineering held in India, and organized by AMPP (formerly known as NACE). The study employs a machine learning tool to identify relationships between spatial variations in internal corrosion.
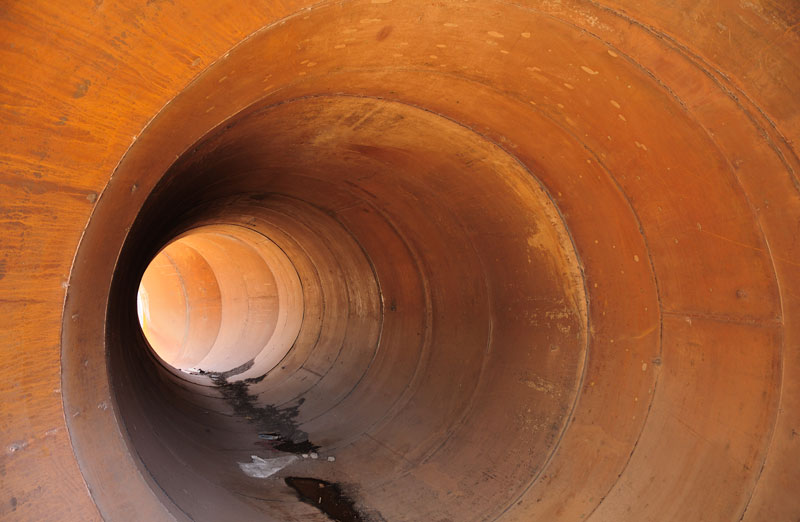
The recycling of pipes used for transporting petroleum products is often hindered by extensive internal corrosion. This corrosion primarily stems from the presence of water, sulfur, and CO2 in the liquid hydrocarbons being transported. In this study, we delve into a particularly corrosive scenario involving the blending of hydrophilic ethanol with naturally sulfur-contaminated gasoline.
Our research aims to achieve two main objectives:
- Projection of Corrosion Susceptibility and Rate: We utilize API 5L pipes to carry an ethanol-gasoline blend, commonly known as gasohol, and project the sections of the pipes prone to corrosion, along with estimating the rate of corrosion.
- Evaluation of Impact on Network Life and Economics: We assess how critical segment corrosion affects the overall lifespan of the pipe network and quantify the resulting economic losses.
To accomplish this, we develop a framework employing Multivariate Adaptive Regression Splines (MARS). This framework allows us to predict pipe corrosion and material loss within a welded (heat-affected) pipe network, which is subjected to internal pressure fatigue in a highly turbulent flow environment.
The model is further simulated using agglomerative clustering to group input variables such as flow conditions, product composition, and the presence of corrosive impurities. Additionally, sensitivity analysis is conducted to understand the effects of design-based variables.
Our results demonstrate a high level of accuracy, with the regressive algorithm achieving over 90% accuracy and a P-value > 0.05 when compared with available physical databases. This tool can be invaluable for engineers and managers, enabling them to estimate thickness loss over time and design cost-effective pipe networks. Furthermore, the model offers insights into potential future adjustments, such as increasing ethanol blend percentages, by checking drop-in requirements based on product composition.
The PPT can accessed from here
,